GNSS Spoofing Detection: Guard against automated ground vehicle attacks
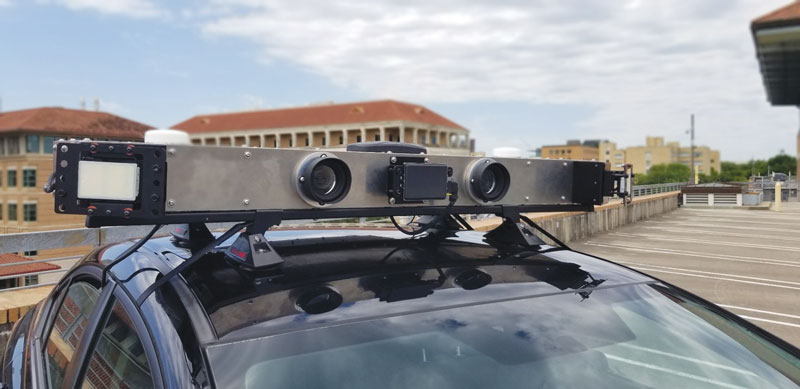
Performance Against a Worst-Case Spoofing Attack. The following is an example of a worst-case spoofing attack in a shallow urban environment. In this scenario, the spoofing attack begins while the vehicle is stopped at a stoplight and continues as the vehicle begins to move. The WFARC in this scenario is shown in Figure 4 with both industrial- and consumer-grade IMUs. The vehicle starts moving at the 163-second mark.
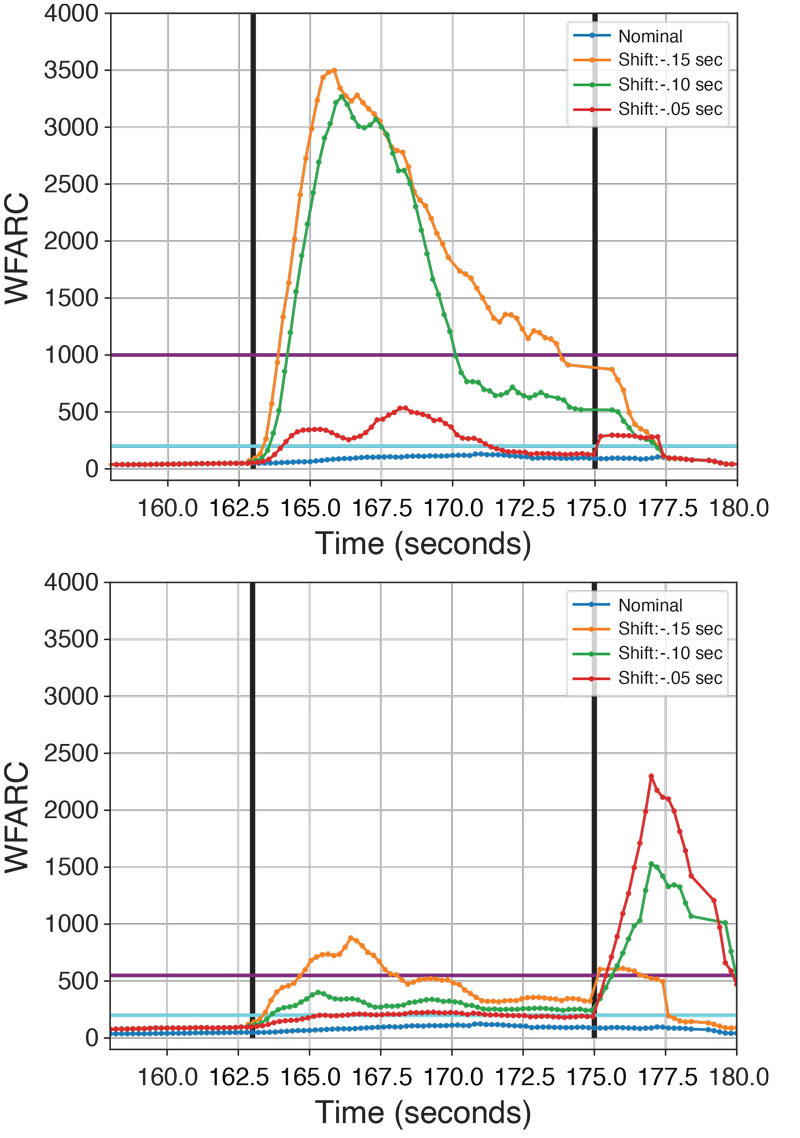
Figure 4: FARC during a worst-case spoofing attack in the shallow urban environment. The top plot is with the industrial grade IMU and the bottom plot is with the consumer grade IMU.
The spoofing attack begins at the 163-second mark just before first movement and ends at the 175-second mark. As the vehicle begins to move, the position errors will grow gradually because the vehicle slowly begins to accelerate forward, inducing a position error.
Three different time-shift attacks in the same scenario are shown in this figure. The shift of 0.15 seconds is the least subtle attack while the 0.05 second attack is the most challenging attack because the faults are much smaller. As the vehicle begins to move, the estimator recognizes inconsistencies between the spoofed GNSS measurements and the IMU because of the tight coupling. The rise in the WFARC above the thresholds shows this disagreement that is attributed to spoofing.
With the industrial-grade IMU and using the shallow urban threshold, all three time shifts spoofing attacks were identified within a second. The estimator knows that the IMU data are different than the GNSS measurements from the WFARC, much more than anything multipath would induce in the shallow urban environment. If the vehicle was in the deep urban environment, the 0.05-second shift spoofing attack would just be attributed to multipath. The sensitivity of the test is dependent on the multipath environment.
All three attacks were identified within two seconds while using the consumer-grade IMU. If the deep urban threshold had been applied, only the least challenging attack would have been identified. In all cases, the WFARC is significantly smaller compared to when the industrial IMU is used. Once again, this is because the estimator has more confidence in the spoofed GNSS measurements than the lower quality IMU.
Interestingly, there is a spike in the WFARC after the spoofing attack is over. This happens because the estimator is showing trauma from the spoofing attack — the abrupt return of the true GNSS measurements, which were significantly different from what the previously ingested spoofed measurements were predicting.
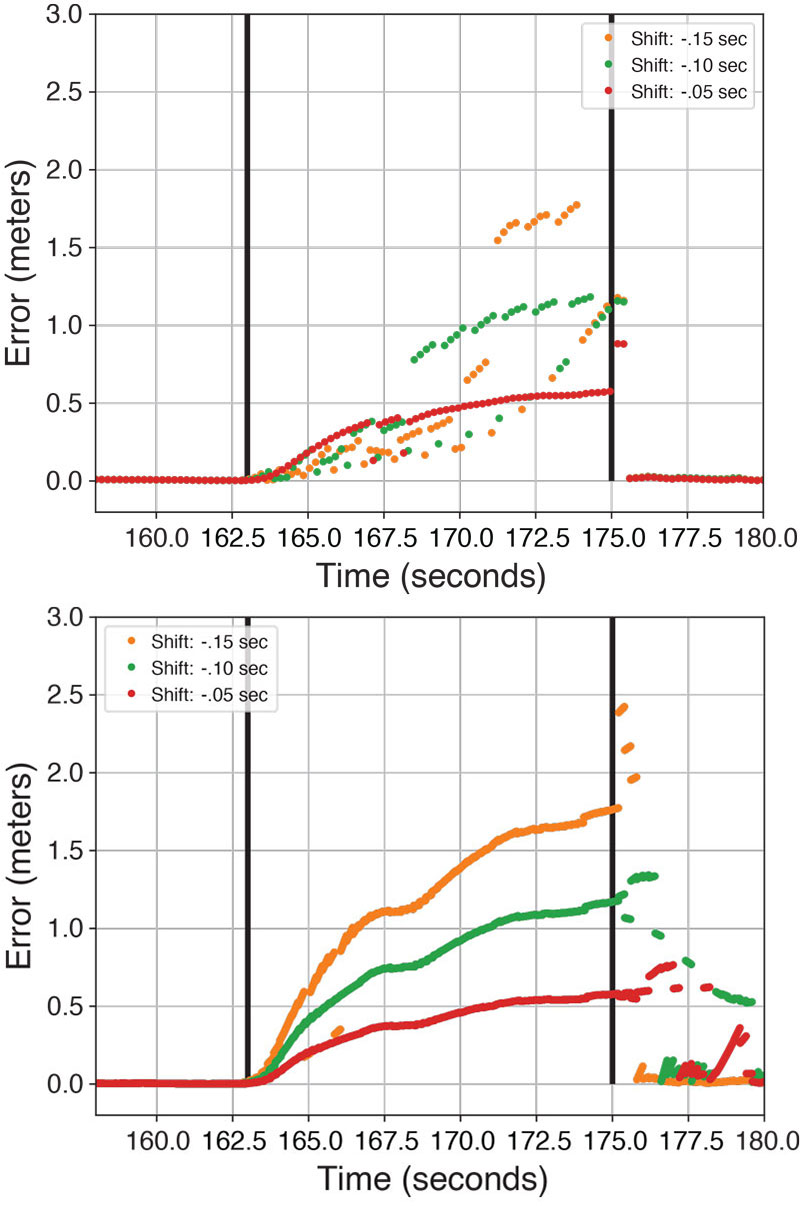
Figure 5: The position errors induced from the different spoofing attacks. The top plot is with the industrial grade IMU and the bottom plot is with the consumer grade IMU.
The corresponding position errors in each attack are shown in Figure 5. The worst-case attack (time shift of –0.05 seconds) only introduces a 0.5 meter offset over 10 seconds, indicative of an extremely subtle attack. Even the least subtle attack (time shift of –0.15 seconds) only introduces a 2-meter offset after 10 seconds, which is much more challenging than the attacks simulated in the related work.

About the Author: Zachary Clements
Zachary Clements (BS, Electrical Engineering, Clemson University) is a graduate student in the Department of Aerospace Engineering, and Engineering Mechanics at The University of Texas (UT) at Austin, and a member of the UT Radionavigation Laboratory.
About the Author: James Yoder
James Yoder (BS, Electrical Engineering, MS, Aerospace Engineering, UT Austin) is a guidance, navigation and control engineer at SpaceX and an alumnus of the UT Radionavigation Laboratory.
Follow Us